AI Integration in Engineering
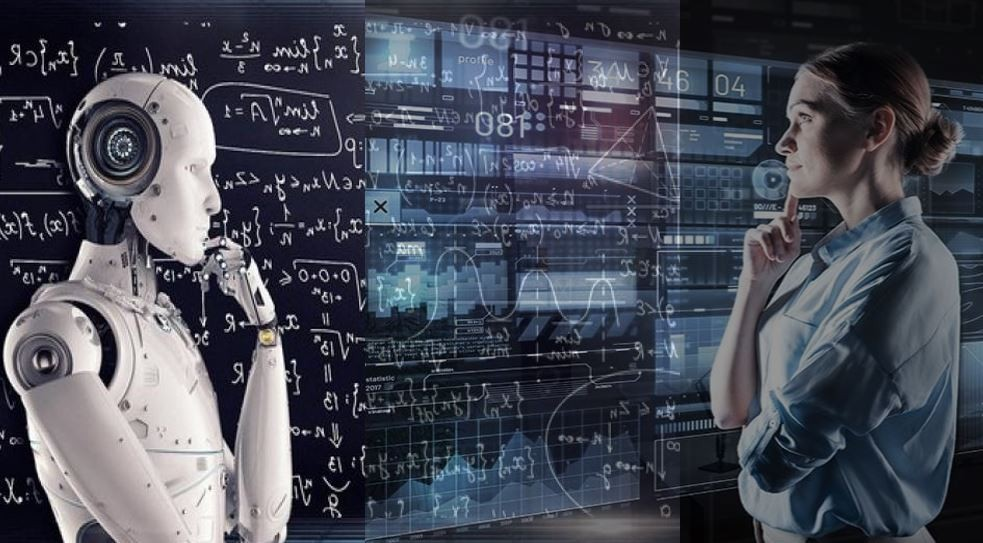
In this era, you must be living under a rock if you've never heard about AI. AI stands for artificial intelligence, which refers to the ability of computers to perform tasks that would typically require humans. A popular example would be ChatGPT, Stockfish, and Midjourny, just to name a few. It is clear that these AIs have surpassed human abilities in their respective areas of expertise. While it may seem scary that they could potentially take over the world, this technological advancement has enabled us to accomplish feats that were previously unimaginable, particularly in the field of engineering. This article will discuss the past and potential integration of AI into the field of engineering.
First, let's define what "engineering" is. Engineering is a field of study that involves the application of mathematics and scientific principles to create machines, systems, or processes. One of its notable accomplishments is the development of steam engines, which, of course, were not AI-integrated. However, in today's world, machines are controlled are computers allowing AI to be integrated.
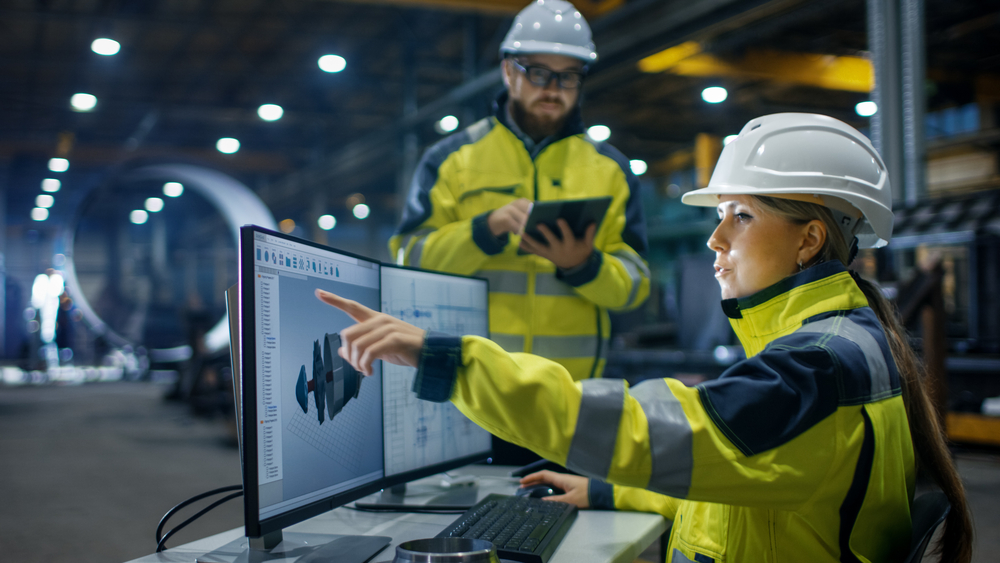
During the early phase of AI integration in the late 1960s, a rule-based or expert system was one of the first to be implemented by industrial engineers. By encoding expert knowledge and rules into the system, it could analyze data from the sensors and measurements to compare them to the standards. We are able to automate things like quality control and process optimization.
Approximately 10 years after the implementation of the rule-based and expert systems into the industry, a new advancement occurred with the invention of machine learning and neural networks. This opened up a new sector in engineering and increased the accuracy and efficiency of quality control through pattern recognition or predictive maintenance, which uses machine learning to forecast equipment failures. It’s important to note that machine learning was not as advanced or widely adopted as it is today.
Fast forward to 2020, and there have been large improvements in machine learning due to advancements in computational power and model complexity. Machine learning (AI) has gained recognition and widespread adoption across various industries. For example, biomedical engineers have adopted the use of image analysis to diagnose patients and predict disease risk. AIs are used to analyze the ECG readings of patients and can accurately diagnose their heart disease and their risk. Furthermore, MIT researchers developed an AI model that is able to detect future lung cancer risks, something that humans can’t accurately do by themselves.
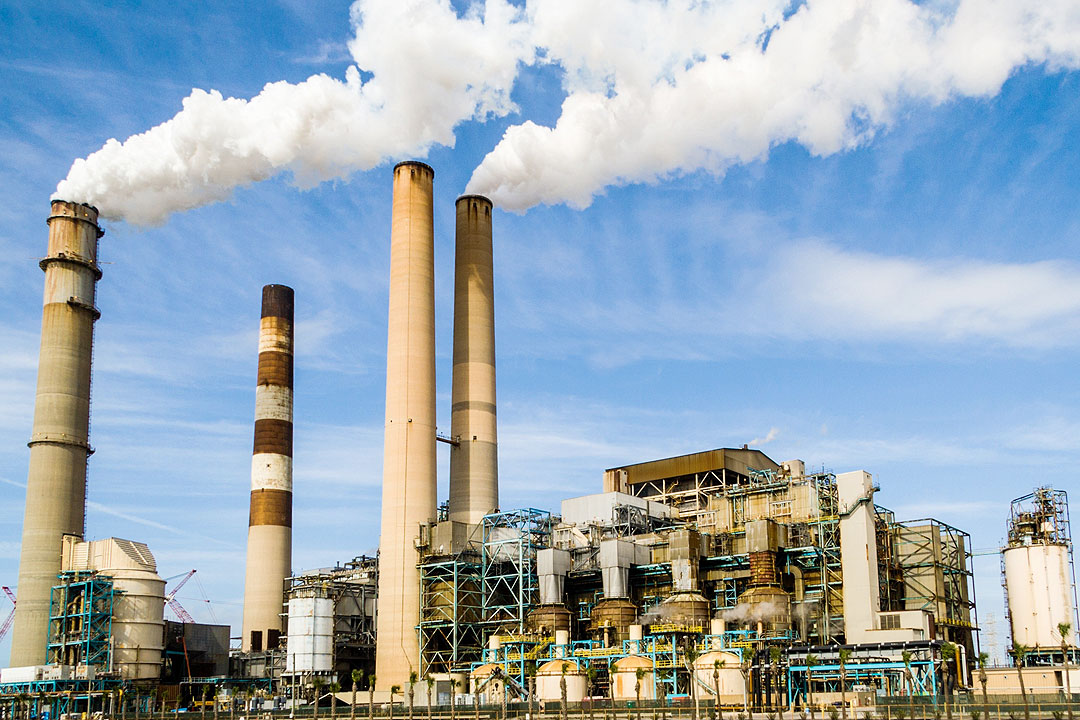
Another sector of engineering that has adopted AI is electrical engineering, where AI is used to increase efficiency, reliability, and sustainability in the electrical sector. AI is used in smart grids to do demand forecasting for load optimization: this involves the AI analyzing past energy consumption and patterns in order for the industry to not over or under-produce. Without AI, it would be much harder to balance power ouput, which could lead to power outages or overproduction of electricity, which wastes energy.
These examples demonstrate how AI has been integrated across various sectors of engineering, bringing improvements in accuracy, efficiency, and sustainability. This may potentially be the new normal for the sector.
It’s to be expected that right now there is a trend toward increasing adoption of AI usage. In the future, many experts believe that there will be a shift in what is expected of engineers, where engineers will in the future have to provide more than their respected field of study.
As of 2023, we have been facing climate problems, and the attention it is gaining is at an all-time-high. Everything from removing garbage from the ocean, to attempts to use tidal waves as a source of energy, to subsidizing electricity from coal is underway to try and solve these pressing issues. Therefore, engineers will have to spread their attention, not just to making things as fast and cheap as possible, but to things such as carbon footprints and GHG emissions. A BCG shows that the use of AI can reduce GHG emissions by 2.6–5.3 gigatonnes of CO2. Engineers will now have to be very conscious of the carbon footprints the indsutry is producing due to the importance of sustainability in this current date and time. Currently, engineers have to track data and find faults in the system, but in the near future, that could all be done using AI. AI would allow engineers to work more effectively by providing them with data and analysis regarding maintenance of the system, tracking carbon footprints, and tracking costs. This would make engineers' lives much easier, and will allow engineers to work purely on knowledge and creativity to find solutions.
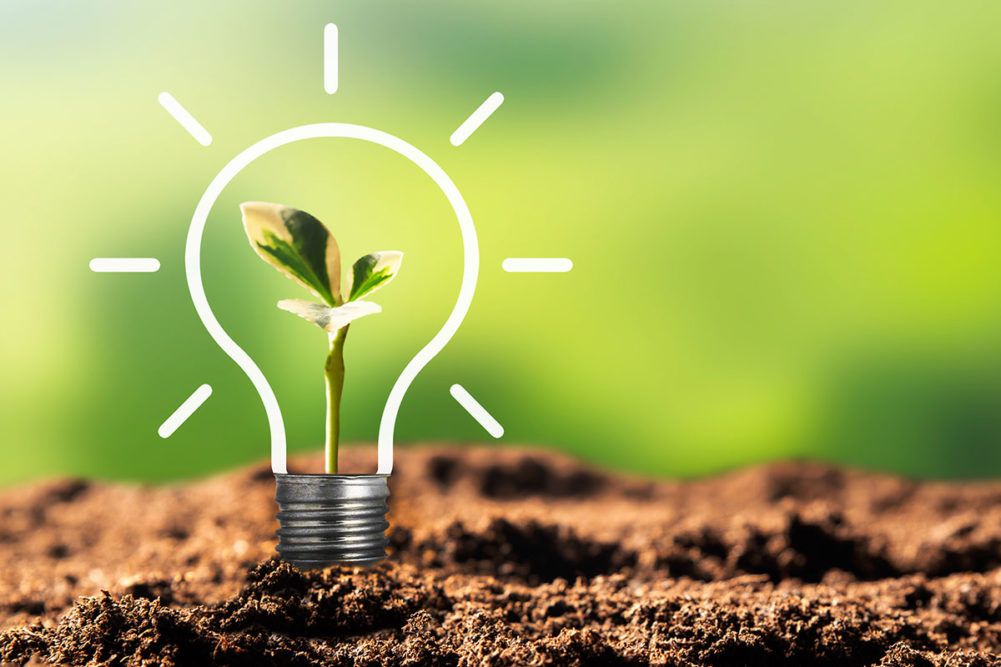
"AI and machine learning are new tools that are not going away, and they are going to help inform engineers on how to do their jobs better," said Professor Jonathan Cagan (Professor, Mechanical Engineering at the College of Engineering, Carnegie Mellon University).
I believe that all current and future engineers, apart from being successful in their sector of expertise, should also learn about the ability and limitations of AI in order to help optimize their productivity as engineers. In the near future, this will be the new normal.